使用者:Siyuwj/沙盒/4

非負矩陣分解(英語:non-negative matrix factorization,NMF),也被稱為非負矩陣估計(英語:non-negative matrix approximation)[1][2]是多元統計分析和線性代數中的一組算法的統稱。這些算法將一個矩陣分解為(通常是)兩個矩陣W和H,三個矩陣都沒有負元素。這一非負性使得最終求解的矩陣更容易被分析。由於這一問題通常不能被準確地求解,因而數值近似方法是常用的求解方法。
非負矩陣分解在計算機視覺、文本聚類[1]、化學計量學和推薦系統中都有應用[3] [4]。
歷史[編輯]
在化學計量學中,非負矩陣分解被稱為「自模式曲線分辨」,由很長的研究歷史[5]。 在這一框架中,右邊矩陣中的向量並非是離散向量,而是連續的曲線。一群芬蘭研究者也曾經在20世紀90年代中期進行過類似的「正矩陣分解」研究工作[6][7]。 當丹尼爾李和塞巴斯蒂安宋研究了這一算法的性質並對兩類分解問題提出了一些簡單有效的算法之後,「非負矩陣分解」這一名稱開始為人熟知[8][9]。
背景[編輯]
使得矩陣V為兩個矩陣W和H的乘積,即
這一矩陣乘積可以實現為將矩陣W的列向量使用矩陣H中的參數進行線性組合得到矩陣V的列向量來計算。亦即,V的每一列都可以用下面的方式來計算:
其中,vi是乘積矩陣V的第i個列向量,hi是乘積矩陣H的第i個列向量。
When multiplying matrices, the dimensions of the factor matrices may be significantly lower than those of the product matrix and it is this property that forms the basis of NMF. NMF generates factors with significantly reduced dimensions compared to the original matrix. For example, if V is an m×n matrix, W is an m×p matrix, and H is a p×n matrix then p can be significantly less than both m and n.
Here's an example based on a text-mining application:
- Let the input matrix (the matrix to be factored) be V with 10000 rows and 500 columns where words are in rows and documents are in columns. That is, we have 500 documents indexed by 10000 words. It follows that a column vector v in V represents a document.
- Assume we ask the algorithm to find 10 features in order to generate a features matrix W with 10000 rows and 10 columns and a coefficients matrix H with 10 rows and 500 columns.
- The product of W and H is a matrix with 10000 rows and 500 columns, the same shape as the input matrix V and, if the factorization worked, also a reasonable approximation to the input matrix V.
- From the treatment of matrix multiplication above it follows that each column in the product matrix WH is a linear combination of the 10 column vectors in the features matrix W with coefficients supplied by the coefficients matrix H.
This last point is the basis of NMF because we can consider each original document in our example as being built from a small set of hidden features. NMF generates these features.
It's useful to think of each feature (column vector) in the features matrix W as a document archetype comprising a set of words where each word's cell value defines the word's rank in the feature: The higher a word's cell value the higher the word's rank in the feature. A column in the coefficients matrix H represents an original document with a cell value defining the document's rank for a feature. This follows because each row in H represents a feature. We can now reconstruct a document (column vector) from our input matrix by a linear combination of our features (column vectors in W where each feature is weighted by the feature's cell value from the document's column in H.
Types[編輯]
Approximate non-negative matrix factorization[編輯]
Usually the number of columns of W and the number of rows of H in NMF are selected so the product WH will become an approximation to V. The full decomposition of V then amounts to the two non-negative matrices W and H as well as a residual U, such that: V = WH + U. The elements of the residual matrix can either be negative or positive.
When W and H are smaller than V they become easier to store and manipulate. Another reason for factorizing V into smaller matrices W and H, is that if one is able to approximately represent the elements of V by significantly less data, then one has to infer some latent structure in the data.
Convex non-negative matrix factorization[編輯]
In standard NMF, matrix factor , i.e., W can be anything in that space. Convex NMF [10] restricts to a be convex combination of the input data vectors . This greatly improves the quality of data representation of W. Furthermore, the resulting matrix factor H becomes more sparse and orthogonal.
Nonnegative rank factorization[編輯]
In case the nonnegative rank of V is equal to its actual rank, V=WH is called a nonnegative rank factorization.[11][12][13] The problem of finding the NRF of V, if it exists, is known to be NP-hard.[14]
Different cost functions and regularizations[編輯]
There are different types of non-negative matrix factorizations. The different types arise from using different cost functions for measuring the divergence between V and WH and possibly by regularization of the W and/or H matrices.[1]
Two simple divergence functions studied by Lee and Seung are the squared error (or Frobenius norm) and an extension of the Kullback–Leibler divergence to positive matrices (the original Kullback–Leibler divergence is defined on probability distributions). Each divergence leads to a different NMF algorithm, usually minimizing the divergence using iterative update rules.
The factorization problem in the squared error version of NMF may be stated as: Given a matrix find nonnegative matrices W and H that minimize the function
Another type of NMF for images is based on the total variation norm.[15]
When L1 regularization (akin to Lasso) is added to NMF with the mean squared error cost function, the resulting problem may be called non-negative sparse coding due to the similarity to the sparse coding problem,[16] although it may also still be referred to as NMF.[17]
Algorithms[編輯]
There are several ways in which the W and H may be found: Lee and Seung's multiplicative update rule [9] has been a popular method due to the simplicity of implementation. Since then, a few other algorithmic approaches have been developed.
Some successful algorithms are based on alternating non-negative least squares: in each step of such an algorithm, first H is fixed and W found by a non-negative least squares solver, then W is fixed and H is found analogously. The procedures used to solve for W and H may be the same[18] or different, as some NMF variants regularize one of W and H.[16] Specific approaches include the projected gradient descent methods,[18][19] the active set method,[3][20] and the block principal pivoting method[21] among several others.
The currently available algorithms are sub-optimal as they can only guarantee finding a local minimum, rather than a global minimum of the cost function. A provably optimal algorithm is unlikely in the near future as the problem has been shown to generalize the k-means clustering problem which is known to be NP-complete.[22] However, as in many other data mining applications, a local minimum may still prove to be useful.
Exact NMF[編輯]
Exact solutions for the variants of NMF can be expected (in polynomial time) when additional constraints hold for matrix V. A polynomial time algorithm for solving nonnegative rank factorization if V contains a monomial sub matrix of rank equal to its rank was given by Campbell and Poole in 1981.[23] Kalofolias and Gallopoulos (2012)[24] solved the symmetric counterpart of this problem, where V is symmetric and contains a diagonal principal sub matrix of rank r. Their algorithm runs in O(rm^2) time in the dense case. Arora, Ge, Halpern, Mimno, Moitra, Sontag, Wu, & Zhu (2013) give a polynomial time algorithm for exact NMF that works for the case where one of the factors W satisfies the separability condition.[25]
Relation to other techniques[編輯]
In Learning the parts of objects by non-negative matrix factorization Lee and Seung proposed NMF mainly for parts-based decomposition of images. It compares NMF to vector quantization and principal component analysis, and shows that although the three techniques may be written as factorizations, they implement different constraints and therefore produce different results.
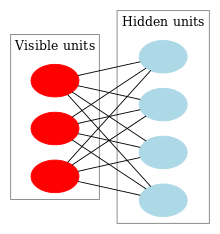
It was later shown that some types of NMF are an instance of a more general probabilistic model called "multinomial PCA".[26] When NMF is obtained by minimizing the Kullback–Leibler divergence, it is in fact equivalent to another instance of multinomial PCA, probabilistic latent semantic analysis,[27] trained by maximum likelihood estimation. That method is commonly used for analyzing and clustering textual data and is also related to the latent class model.
It has been shown [28][29] NMF is equivalent to a relaxed form of K-means clustering: matrix factor W contains cluster centroids and H contains cluster membership indicators, when using the least square as NMF objective. This provides theoretical foundation for using NMF for data clustering.
NMF can be seen as a two-layer directed graphical model with one layer of observed random variables and one layer of hidden random variables.[30]
NMF extends beyond matrices to tensors of arbitrary order.[31][32][33] This extension may be viewed as a non-negative version of, e.g., the PARAFAC model.
Other extensions of NMF include joint factorisation of several data matrices and tensors where some factors are shared. Such models are useful for sensor fusion and relational learning.[34]
NMF is an instance of the nonnegative quadratic programming (NQP) as well as many other important problems including the support vector machine (SVM). However, SVM and NMF are related at a more intimate level than that of NQP, which allows direct application of the solution algorithms developed for either of the two methods to problems in both domains.[35]
Uniqueness[編輯]
The factorization is not unique: A matrix and its inverse can be used to transform the two factorization matrices by, e.g.,[36]
If the two new matrices and are non-negative they form another parametrization of the factorization.
The non-negativity of and applies at least if B is a non-negative monomial matrix. In this simple case it will just correspond to a scaling and a permutation.
More control over the non-uniqueness of NMF is obtained with sparsity constraints.[37]
Clustering property[編輯]
NMF has an inherent clustering property,[28] i.e., it automatically clusters the columns of input data .
More specifically, the approximation of by is achieved by minimizing the error function
subject to
If we add additional orthogonality constraint on , i.e., , then the above minimization is identical to the minimization of K-means clustering.
Furthermore, the computed gives the cluster indicator, i.e., if , that fact indicates input data belongs/assigned to cluster. And the computed gives the cluster centroids, i.e., the column gives the cluster centroid of cluster.
When the orthogonality is not explicitly imposed, the orthogonality holds to a large extent, and the clustering property holds too, as in most applications of NMF.
When the error function is replaced by Kullback–Leibler divergence, it is proved [38] shown that NMF is identical to the Probabilistic latent semantic analysis, a popular document clustering method.
Applications[編輯]
Text mining[編輯]
NMF can be used for text mining applications. In this process, a document-term matrix is constructed with the weights of various terms (typically weighted word frequency information) from a set of documents. This matrix is factored into a term-feature and a feature-document matrix. The features are derived from the contents of the documents, and the feature-document matrix describes data clusters of related documents.
One specific application used hierarchical NMF on a small subset of scientific abstracts from PubMed.[39] Another research group clustered parts of the Enron email dataset[40] with 65,033 messages and 91,133 terms into 50 clusters.[41] NMF has also been applied to citations data, with one example clustering Wikipedia articles and scientific journals based on the outbound scientific citations in Wikipedia.[42]
Arora, Ge, Halpern, Mimno, Moitra, Sontag, Wu, & Zhu (2013) have given polynomial-time algorithms to learn topic models using NMF. The algorithm assumes that the topic matrix satisfies a separability condition that is often found to hold in these settings. [25]
Spectral data analysis[編輯]
NMF is also used to analyze spectral data; one such use is in the classification of space objects and debris.[43]
Scalable Internet distance prediction[編輯]
NMF is applied in scalable Internet distance (round-trip time) prediction. For a network with hosts, with the help of NMF, the distances of all the end-to-end links can be predicted after conducting only measurements. This kind of method was firstly introduced in Internet Distance Estimation Service (IDES).[44] Afterwards, as a fully decentralized approach, Phoenix network coordinate system [45] is proposed. It achieves better overall prediction accuracy by introducing the concept of weight.
Non-stationary speech denoising[編輯]
Speech denoising has been a long lasting problem in audio signal processing. There are lots of algorithms for denoising if the noise is stationary. For example, the Wiener filter is suitable for additive Gaussian noise. However, if the noise is non-stationary, the classical denoising algorithms usually have poor performance because the statistical information of the non-stationary noise is difficult to estimate. Schmidt et al.[46] use NMF to do speech denoising under non-stationary noise, which is completely different from classical statistical approaches.The key idea is that clean speech signal can be sparsely represented by a speech dictionary, but non-stationary noise cannot. Similarly, non-stationary noise can also be sparsely represented by a noise dictionary, but speech cannot.
The algorithm for NMF denoising goes as follows. Two dictionaries, one for speech and one for noise, need to be trained offline. Once a noisy speech is given, we first calculate the magnitude of the Short-Time-Fourier-Transform. Second, separate it into two parts via NMF, one can be sparsely represented by the speech dictionary, and the other part can be sparsely represented by the noise dictionary. Third, the part that is represented by the speech dictionary will be the estimated clean speech.
Bioinformatics[編輯]
NMF has been successfully applied to bioinformatics.[47][48]
Current research[編輯]
Current[何時?] research in nonnegative matrix factorization includes, but not limited to,
(1) Algorithmic: searching for global minima of the factors and factor initialization.[49]
(2) Scalability: how to factorize million-by-billion matrices, which are commonplace in Web-scale data mining, e.g., see Distributed Nonnegative Matrix Factorization (DNMF)[50]
(3) Online: how to update the factorization when new data comes in without recomputing from scratch.
See also[編輯]
- Online NMF (Online non-negative matrix factorization)
- Multilinear algebra
- Multilinear subspace learning
- Tensor
- Tensor decomposition
- Tensor software
Sources and external links[編輯]
Notes[編輯]
- ^ 1.0 1.1 1.2 Inderjit S. Dhillon, Suvrit Sra. Generalized Nonnegative Matrix Approximations with Bregman Divergences (PDF). NIPS. 2005.
- ^ Tandon, Rashish; Suvrit Sra. Sparse nonnegative matrix approximation: new formulations and algorithms (PDF). TR. 2010.
- ^ 3.0 3.1 Rainer Gemulla, Erik Nijkamp, Peter J Haas, Yannis Sismanis. Large-scale matrix factorization with distributed stochastic gradient descent (PDF). Proc. ACM SIGKDD Int'l Conf. on Knowledge discovery and data mining: 69–77. 2011.
- ^ Yang Bao et al. TopicMF: Simultaneously Exploiting Ratings and Reviews for Recommendation. AAAI. 2014.
- ^ William H. Lawton; Edward A. Sylvestre. Self modeling curve resolution. Technometrics. 1971, 13 (3): 617+. doi:10.2307/1267173.
- ^ P. Paatero, U. Tapper. Positive matrix factorization: A non-negative factor model with optimal utilization of error estimates of data values. Environmetrics. 1994, 5 (2): 111–126. doi:10.1002/env.3170050203.
- ^ Pia Anttila, Pentti Paatero, Unto Tapper, Olli Järvinen. Source identification of bulk wet deposition in Finland by positive matrix factorization. Atmospheric Environment. 1995, 29 (14): 1705–1718. doi:10.1016/1352-2310(94)00367-T.
- ^ 8.0 8.1 Daniel D. Lee and H. Sebastian Seung. Learning the parts of objects by non-negative matrix factorization. Nature. 1999, 401 (6755): 788–791. PMID 10548103. doi:10.1038/44565.
- ^ 9.0 9.1 Daniel D. Lee and H. Sebastian Seung. Algorithms for Non-negative Matrix Factorization. Advances in Neural Information Processing Systems 13: Proceedings of the 2000 Conference. MIT Press: 556–562. 2001.
- ^ C Ding, T Li, MI Jordan, Convex and semi-nonnegative matrix factorizations, IEEE Transactions on Pattern Analysis and Machine Intelligence, 32, 45-55, 2010
- ^ Berman, A.; R.J. Plemmons. Inverses of nonnegative matrices. Linear and Multilinear Algebra. 1974, 2: 161–172. doi:10.1080/03081087408817055.
- ^ A. Berman, R.J. Plemmons. Nonnegative matrices in the Mathematical Sciences. Philadelphia: SIAM. 1994.
- ^ Thomas, L.B. Problem 73-14, Rank factorization of nonnegative matrices. SIAM rev. 1974, 16 (3): 393–394. doi:10.1137/1016064.
- ^ Vavasis, S.A. On the complexity of nonnegative matrix factorization. SIAM J. Optim. 2009, 20: 1364–1377. doi:10.1137/070709967.
- ^ Taiping Zhang, Bin Fang, Weining Liu, Yuan Yan Tang, Guanghui He, Jing Wen. Total variation norm-based nonnegative matrix factorization for identifying discriminant representation of image patterns. Neurocomputing. 2008-06, 71 (10-12): 1824–1831 [2019-06-25]. doi:10.1016/j.neucom.2008.01.022 (英語).
- ^ 16.0 16.1 Hoyer, Patrik O. Non-negative sparse coding. Proc. IEEE Workshop on Neural Networks for Signal Processing. 2002.
- ^ Cho-Jui Hsieh, Inderjit S. Dhillon. Fast coordinate descent methods with variable selection for non-negative matrix factorization. ACM Press: 1064. 2011 [2019-06-25]. ISBN 9781450308137. doi:10.1145/2020408.2020577 (英語).
- ^ 18.0 18.1 Chih-Jen Lin. Projected Gradient Methods for Nonnegative Matrix Factorization. Neural Computation. 2007-10, 19 (10): 2756–2779 [2019-06-25]. ISSN 0899-7667. doi:10.1162/neco.2007.19.10.2756 (英語).
- ^ Chih-Jen Lin. On the Convergence of Multiplicative Update Algorithms for Nonnegative Matrix Factorization. IEEE Transactions on Neural Networks. 2007-11, 18 (6): 1589–1596 [2019-06-25]. ISSN 1045-9227. doi:10.1109/TNN.2007.895831.
- ^ Hyunsoo Kim and Haesun Park. Nonnegative Matrix Factorization Based on Alternating Nonnegativity Constrained Least Squares and Active Set Method (PDF). SIAM Journal on Matrix Analysis and Applications. 2008, 30 (2): 713–730. doi:10.1137/07069239x.
- ^ Jingu Kim and Haesun Park. Fast Nonnegative Matrix Factorization: An Active-set-like Method and Comparisons (PDF). SIAM Journal on Scientific Computing. 2011, 33 (6): 3261–3281. doi:10.1137/110821172.
- ^ Ding, C. and He, X. and Simon, H.D.,. On the equivalence of nonnegative matrix factorization and spectral clustering. Proc. SIAM Data Mining Conf. 2005, 4: 606–610. doi:10.1137/1.9781611972757.70.
- ^ Campbell, S.L.; G.D. Poole. Computing nonnegative rank factorizations.. Linear Algebra Appl. 1981, 35: 175–182. doi:10.1016/0024-3795(81)90272-x.
- ^ Kalofolias, V.; Gallopoulos, E. Computing symmetric nonnegative rank factorizations. Linear Algebra Appl. 2012, 436: 421–435. doi:10.1016/j.laa.2011.03.016.
- ^ 25.0 25.1 Arora, Sanjeev; Ge, Rong; Halpern, Yoni; Mimno, David; Moitra, Ankur; Sontag, David; Wu, Yichen; Zhu, Michael. A practical algorithm for topic modeling with provable guarantees. Proceedings of the 30th International Conference on Machine Learning. 2013. arXiv:1212.4777
.
- ^ Wray Buntine. Variational Extensions to EM and Multinomial PCA (PDF). Proc. European Conference on Machine Learning (ECML-02). LNAI 2430: 23–34. 2002.
- ^ Eric Gaussier and Cyril Goutte. Relation between PLSA and NMF and Implications (PDF). Proc. 28th international ACM SIGIR conference on Research and development in information retrieval (SIGIR-05): 601–602. 2005.
- ^ 28.0 28.1 C. Ding, X. He, H.D. Simon (2005). "On the Equivalence of Nonnegative Matrix Factorization and Spectral Clustering". Proc. SIAM Int'l Conf. Data Mining, pp. 606-610. May 2005
- ^ Ron Zass and Amnon Shashua (2005). "A Unifying Approach to Hard and Probabilistic Clustering". International Conference on Computer Vision (ICCV) Beijing, China, Oct., 2005.
- ^ Max Welling et al. Exponential Family Harmoniums with an Application to Information Retrieval. NIPS. 2004.
- ^ Pentti Paatero. The Multilinear Engine: A Table-Driven, Least Squares Program for Solving Multilinear Problems, including the n-Way Parallel Factor Analysis Model. Journal of Computational and Graphical Statistics. 1999, 8 (4): 854–888. JSTOR 1390831. doi:10.2307/1390831.
- ^ Max Welling and Markus Weber. Positive Tensor Factorization. Pattern Recognition Letters. 2001, 22 (12): 1255–1261. doi:10.1016/S0167-8655(01)00070-8.
- ^ Jingu Kim and Haesun Park. Fast Nonnegative Tensor Factorization with an Active-set-like Method (PDF). High-Performance Scientific Computing: Algorithms and Applications. Springer: 311–326. 2012.
- ^ Kenan Yilmaz and A. Taylan Cemgil and Umut Simsekli. Generalized Coupled Tensor Factorization (PDF). NIPS. 2011.
- ^ Vamsi K. Potluru and Sergey M. Plis and Morten Morup and Vince D. Calhoun and Terran Lane. Efficient Multiplicative updates for Support Vector Machines. Proceedings of the 2009 SIAM Conference on Data Mining (SDM): 1218–1229. 2009.
- ^ Wei Xu, Xin Liu & Yihong Gong. Document clustering based on non-negative matrix factorization. Proceedings of the 26th annual international ACM SIGIR conference on Research and development in information retrieval. New York: Association for Computing Machinery: 267–273. 2003.
- ^ Julian Eggert, Edgar Körner, "Sparse coding and NMF", Proceedings. 2004 IEEE International Joint Conference on Neural Networks, 2004, pp. 2529-2533, 2004.
- ^ C Ding, T Li, W Peng, " On the equivalence between non-negative matrix factorization and probabilistic latent semantic indexing" Computational Statistics & Data Analysis 52, 3913-3927
- ^ Nielsen, Finn Årup; Balslev, Daniela; Hansen, Lars Kai. Mining the posterior cingulate: segregation between memory and pain components. NeuroImage. 2005, 27 (3): 520–522. PMID 15946864. doi:10.1016/j.neuroimage.2005.04.034.
- ^ Cohen, William. Enron Email Dataset. 2005-04-04 [2008-08-26].
- ^ Berry, Michael W.; Browne, Murray. Email Surveillance Using Non-negative Matrix Factorization. Computational and Mathematical Organization Theory. 2005, 11 (3): 249–264. doi:10.1007/s10588-005-5380-5.
- ^ Nielsen, Finn Årup. Clustering of scientific citations in Wikipedia. Wikimania. 2008.
- ^ Michael W. Berry; et al. Algorithms and Applications for Approximate Nonnegative Matrix Factorization. 2006.
- ^ Yun Mao, Lawrence Saul and Jonathan M. Smith. IDES: An Internet Distance Estimation Service for Large Networks. IEEE Journal on Selected Areas in Communications. 2006, 24 (12): 2273–2284. doi:10.1109/JSAC.2006.884026.
- ^ Yang Chen, Xiao Wang, Cong Shi, and; et al. Phoenix: A Weight-based Network Coordinate System Using Matrix Factorization (PDF). IEEE Transactions on Network and Service Management. 2011, 8 (4): 334–347. doi:10.1109/tnsm.2011.110911.100079.
- ^ Schmidt, M.N., J. Larsen, and F.T. Hsiao. (2007). "Wind noise reduction using non-negative sparse coding", Machine Learning for Signal Processing, IEEE Workshop on, 431–436
- ^ Devarajan, K. Nonnegative Matrix Factorization: An Analytical and Interpretive Tool in Computational Biology. PLoS Computational Biology. 2008, 4 (7).
- ^ Hyunsoo Kim and Haesun Park. Sparse non-negative matrix factorizations via alternating non-negativity-constrained least squares for microarray data analysis. Bioinformatics. 2007, 23 (12): 1495–1502. PMID 17483501. doi:10.1093/bioinformatics/btm134.
- ^ C. Boutsidis and E. Gallopoulos. SVD based initialization: A head start for nonnegative matrix factorization. Pattern Recognition. 2008, 41 (4): 1350–1362. doi:10.1016/j.patcog.2007.09.010.
- ^ Chao Liu, Hung-chih Yang, Jinliang Fan, Li-Wei He, and Yi-Min Wang. Distributed Nonnegative Matrix Factorization for Web-Scale Dyadic Data Analysis on MapReduce (PDF). Proceedings of the 19th International World Wide Web Conference. 2010.
Others[編輯]
- J. Shen, G. W. Israël. A receptor model using a specific non-negative transformation technique for ambient aerosol. Atmospheric Environment. 1989, 23 (10): 2289–2298. doi:10.1016/0004-6981(89)90190-X.
- Pentti Paatero. Least squares formulation of robust non-negative factor analysis. Chemometrics and Intelligent Laboratory Systems. 1997, 37 (1): 23–35. doi:10.1016/S0169-7439(96)00044-5.
- Raul Kompass. A Generalized Divergence Measure for Nonnegative Matrix Factorization. Neural Computation. 2007, 19 (3): 780–791. PMID 17298233. doi:10.1162/neco.2007.19.3.780.
- Liu, W.X. and Zheng, N.N. and You, Q.B. Nonnegative Matrix Factorization and its applications in pattern recognition (PDF). Chinese Science Bulletin. 2006, 51 (17–18): 7–18. doi:10.1007/s11434-005-1109-6.
- Ngoc-Diep Ho, Paul Van Dooren and Vincent Blondel. Descent Methods for Nonnegative Matrix Factorization. 2008. arXiv:0801.3199
[cs.NA].
- Andrzej Cichocki, Rafal Zdunek and Shun-ichi Amari. Nonnegative Matrix and Tensor Factorization. IEEE Signal Processing Magazine. 2008, 25 (1): 142–145. doi:10.1109/MSP.2008.4408452.
- Cédric Févotte, Nancy Bertin, and Jean-Louis Durrieu. Nonnegative Matrix Factorization with the Itakura-Saito Divergence: With Application to Music Analysis. Neural Computation. 2009, 21 (3): 793–830. PMID 18785855. doi:10.1162/neco.2008.04-08-771.
- Ali Taylan Cemgil. Bayesian Inference for Nonnegative Matrix Factorisation Models. Computational Intelligence and Neuroscience. 2009, 2009 (2): 1. PMC 2688815
. PMID 19536273. doi:10.1155/2009/785152.
[[Category:线性代数]] [[Category:矩阵理论]] [[Category:多元统计]] [[Category:机器学习]]