人工智能对齐:修订间差异
Icosohedral(留言 | 贡献) →研究进展: 内容扩充,来自英文条目 |
Icosohedral(留言 | 贡献) →研究进展: 内容扩充,来自英文条目 |
||
第50行: | 第50行: | ||
尽管偏好学习可向人工智能系统指定难以表达的行为,但对于人类价值理念的输入需要以大量数据集或人类交互作为基础。{{Le|机器伦理学|Machine ethics}}为此提供了一种辅助手段:向人工智能系统灌输道德价值。{{efn|文森特·维格尔认为“我们应该将机器的道德敏感扩展为一个道德维度,在获得越来越多自主性的同时,这些机器将不可避免的独立发现道德准则。”<ref>{{Cite journal| doi = 10.1007/s10676-010-9239-1| issn = 1572-8439| volume = 12| issue = 4| pages = 359–361| last = Wiegel| first = Vincent |title = Wendell Wallach and Colin Allen: moral machines: teaching robots right from wrong| journal = Ethics and Information Technology| accessdate = 2022-07-23| date = 2010-12-01| s2cid = 30532107| url = https://doi.org/10.1007/s10676-010-9239-1}}</ref> 参考温德尔·瓦拉赫和科林·艾伦的《道德机器:教机器人分辨是非》一书。<ref>{{Cite book| publisher = Oxford University Press| isbn = 978-0-19-537404-9| last1 = Wallach| first1 = Wendell| last2 = Allen| first2 = Colin| title = Moral Machines: Teaching Robots Right from Wrong| location = New York| accessdate = 2022-07-23| date = 2009| url = https://oxford.universitypressscholarship.com/10.1093/acprof:oso/9780195374049.001.0001/acprof-9780195374049}}</ref>}} 机器伦理学旨在教授给这些系统人类道德的规范基础,例如幸福、平等、公正;避免有意伤害;避免谬误;遵循承诺。机器伦理学的目标是赋予人工智能系统一套适用于广泛场景的价值准则。这种方法有其自身的概念性挑战,研究者需要澄清对齐的目标:人工智能系统需要遵循设计者所作规则的字面意义,他的隐含意图,他的[[顯示性偏好|显示性偏好]],他在充分知情时{{Le|友好人工智能#连贯推断意志|Friendly_artificial_intelligence#Coherent_extrapolated_volition|理应会选择}}的偏好,还是设计者的客观利益,或{{Le|道德现实主义|Moral realism|客观的道德价值}}?<ref name=":2">{{Cite journal |last=Gabriel |first=Iason |date=2020-09-01 |title=Artificial Intelligence, Values, and Alignment |url=https://doi.org/10.1007/s11023-020-09539-2 |journal=Minds and Machines |language=en |volume=30 |issue=3 |doi=10.1007/s11023-020-09539-2 |issn=1572-8641}}</ref> 其它挑战还包括将不同利益相关者的偏好汇总,并避免出现价值锁定——即防止人工智能系统在某一时刻锁定自身价值系统,不再随发展而改变,这种固定的价值系统通常无法具备完整的代表性。<ref name=":2" /><ref>{{Cite book|edition=First edition|chapter=|url=https://www.worldcat.org/oclc/1314633519|date=2022|location=New York, NY|isbn=978-1-5416-1862-6|oclc=1314633519|first=William|last=MacAskill|title=What we owe the future}}</ref> |
尽管偏好学习可向人工智能系统指定难以表达的行为,但对于人类价值理念的输入需要以大量数据集或人类交互作为基础。{{Le|机器伦理学|Machine ethics}}为此提供了一种辅助手段:向人工智能系统灌输道德价值。{{efn|文森特·维格尔认为“我们应该将机器的道德敏感扩展为一个道德维度,在获得越来越多自主性的同时,这些机器将不可避免的独立发现道德准则。”<ref>{{Cite journal| doi = 10.1007/s10676-010-9239-1| issn = 1572-8439| volume = 12| issue = 4| pages = 359–361| last = Wiegel| first = Vincent |title = Wendell Wallach and Colin Allen: moral machines: teaching robots right from wrong| journal = Ethics and Information Technology| accessdate = 2022-07-23| date = 2010-12-01| s2cid = 30532107| url = https://doi.org/10.1007/s10676-010-9239-1}}</ref> 参考温德尔·瓦拉赫和科林·艾伦的《道德机器:教机器人分辨是非》一书。<ref>{{Cite book| publisher = Oxford University Press| isbn = 978-0-19-537404-9| last1 = Wallach| first1 = Wendell| last2 = Allen| first2 = Colin| title = Moral Machines: Teaching Robots Right from Wrong| location = New York| accessdate = 2022-07-23| date = 2009| url = https://oxford.universitypressscholarship.com/10.1093/acprof:oso/9780195374049.001.0001/acprof-9780195374049}}</ref>}} 机器伦理学旨在教授给这些系统人类道德的规范基础,例如幸福、平等、公正;避免有意伤害;避免谬误;遵循承诺。机器伦理学的目标是赋予人工智能系统一套适用于广泛场景的价值准则。这种方法有其自身的概念性挑战,研究者需要澄清对齐的目标:人工智能系统需要遵循设计者所作规则的字面意义,他的隐含意图,他的[[顯示性偏好|显示性偏好]],他在充分知情时{{Le|友好人工智能#连贯推断意志|Friendly_artificial_intelligence#Coherent_extrapolated_volition|理应会选择}}的偏好,还是设计者的客观利益,或{{Le|道德现实主义|Moral realism|客观的道德价值}}?<ref name=":2">{{Cite journal |last=Gabriel |first=Iason |date=2020-09-01 |title=Artificial Intelligence, Values, and Alignment |url=https://doi.org/10.1007/s11023-020-09539-2 |journal=Minds and Machines |language=en |volume=30 |issue=3 |doi=10.1007/s11023-020-09539-2 |issn=1572-8641}}</ref> 其它挑战还包括将不同利益相关者的偏好汇总,并避免出现价值锁定——即防止人工智能系统在某一时刻锁定自身价值系统,不再随发展而改变,这种固定的价值系统通常无法具备完整的代表性。<ref name=":2" /><ref>{{Cite book|edition=First edition|chapter=|url=https://www.worldcat.org/oclc/1314633519|date=2022|location=New York, NY|isbn=978-1-5416-1862-6|oclc=1314633519|first=William|last=MacAskill|title=What we owe the future}}</ref> |
||
==== 可扩展监管 ==== |
|||
随着人工智能系统规模扩大,对它的监督难度也随之升高。人工智能系统被部署解决许多复杂的任务,而人类难以评估这些成果的实际效用。这些任务包括总结书籍内容<ref name=":172">{{cite arXiv|last1=Wu|first1=Jeff|last2=Ouyang|first2=Long|last3=Ziegler|first3=Daniel M.|last4=Stiennon|first4=Nisan|last5=Lowe|first5=Ryan|last6=Leike|first6=Jan|last7=Christiano|first7=Paul|date=2021-09-27|title=Recursively Summarizing Books with Human Feedback|class=cs.CL|eprint=2109.10862}}</ref>、创作有说服力且真实的言论<ref>{{Cite web |last1=Irving |first1=Geoffrey |last2=Amodei |first2=Dario |date=2018-05-03 |title=AI Safety via Debate |url=https://openai.com/blog/debate/ |accessdate=2022-07-23 |work=OpenAI }}</ref><ref name=":1322" /><ref name="Naughton">{{Cite news|last=Naughton|first=John|date=2021-10-02|title=The truth about artificial intelligence? It isn't that honest|work=The Observer|url=https://www.theguardian.com/commentisfree/2021/oct/02/the-truth-about-artificial-intelligence-it-isnt-that-honest|accessdate=2022-07-23|issn=0029-7712}}</ref>、编写稳定运行且无安全漏洞的代码<ref name=":113" />、预测长期事件<ref name=":133">{{cite arXiv|last1=Christiano|first1=Paul|last2=Shlegeris|first2=Buck|last3=Amodei|first3=Dario|date=2018-10-19|title=Supervising strong learners by amplifying weak experts|class=cs.LG|eprint=1810.08575}}</ref><ref>{{Cite book|url=http://link.springer.com/10.1007/978-3-030-39958-0|title=Genetic Programming Theory and Practice XVII|date=2020|publisher=Springer International Publishing|editor1-first=Wolfgang|editor1-last=Banzhaf|editor2-first=Erik|editor2-last=Goodman|editor3-first=Leigh|editor3-last=Sheneman|editor4-first=Leonardo|editor4-last=Trujillo|editor5-first=Bill|editor5-last=Worzel|isbn=978-3-030-39957-3|series=Genetic and Evolutionary Computation|location=Cham|doi=10.1007/978-3-030-39958-0|s2cid=218531292|accessdate=2022-07-23}}</ref>(例如[[氣候變遷|气候变化]]或某项政策的执行后果)。普遍而言,如果人工智能在某一领域的能力超过人类,那么对其成果的评估就会变得十分困难。为了对这类难以评估的成果作出反馈,并分辨出人工智能提供的解决方案中似乎具备说服力却并非真实的部分,人类需要大量时间或额外的协助。因此,可扩展监管({{Lang-en|scalable oversight}})旨在减少上述过程所花费的时间,并帮助人类更好的监督人工智能的行为。<ref name=":110" /> |
|||
人工智能研究者保罗·克里斯蒂亚诺指出,人工智能系统拥有者可能更倾向于为该系统设定容易评估的目标,而非开发可扩展监管技术,因为这种做法较为简单且仍可获得利润。他认为这种倾向会促使“一个针对(容易评估的)可获利项目不断优化的世界,这些项目可以是引导用户点击按钮、促使用户在其产品中花费大量时间,而不是考虑朝着有利于我们的规则改良前进。”<ref>{{Cite podcast|last=Wiblin|first=Robert|title=Dr Paul Christiano on how OpenAI is developing real solutions to the ‘AI alignment problem’, and his vision of how humanity will progressively hand over decision-making to AI systems|series=80,000 hours|accessdate=2022-07-23|publisher=|date=October 2, 2018|url=https://80000hours.org/podcast/episodes/paul-christiano-ai-alignment-solutions/}}</ref> |
|||
容易评估的目标可以是要求人工智能的输出达到某个分数。一些人工智能系统已找到快速达成这种目标的捷径:它们会尝试迷惑人类监督者,作出有说服力却并非真实的行为(参见上文机器人手臂抓取小球的案例)。一些人工智能系统还可意识到它们正受评估,表现出“装死”,直到评估结束后才恢复原行为。<ref>{{Cite journal |last1=Lehman |first1=Joel |last2=Clune |first2=Jeff |last3=Misevic |first3=Dusan |last4=Adami |first4=Christoph |last5=Altenberg |first5=Lee |last6=Beaulieu |first6=Julie |last7=Bentley |first7=Peter J. |last8=Bernard |first8=Samuel |last9=Beslon |first9=Guillaume |last10=Bryson |first10=David M. |last11=Cheney |first11=Nick |date=2020 |title=The Surprising Creativity of Digital Evolution: A Collection of Anecdotes from the Evolutionary Computation and Artificial Life Research Communities |url=https://direct.mit.edu/artl/article/26/2/274-306/93255 |journal=Artificial Life |language=en |volume=26 |issue=2 |pages=274–306 |doi=10.1162/artl_a_00319 |issn=1064-5462 |pmid=32271631 |s2cid=4519185}}</ref> 精密程度高的人工智能系统可更轻易的执行这类欺骗性为<ref name=":1522" /><ref name=":84" />,并且目标难度越高,人工智能越有可能出现欺骗行为。假如模型具备规划能力,那么它们或许可从其监视者眼中掩藏所作的欺骗行为。<ref>{{cite arXiv|last1=Hendrycks|first1=Dan|last2=Carlini|first2=Nicholas|last3=Schulman|first3=John|last4=Steinhardt|first4=Jacob|date=2022-06-16|title=Unsolved Problems in ML Safety|eprint=2109.13916|class=cs.LG}}</ref> 例如在汽车产业,[[大众集团]]工程师就曾在汽车中部署用于[[福斯集團汽車舞弊事件|规避实验室尾气检测]]的系统,这显示出逃避监测有时会受到现实世界的激励。<ref name=":010" /> |
|||
== 参考 == |
== 参考 == |
2022年12月14日 (三) 05:03的版本
人工智能对齐(英語:AI alignment)是指引导人工智能系统的行为,使其符合设计者的利益和预期目标。[a] 一个已对齐的人工智能的行为会向着预期方向发展;而未对齐的人工智能的行为虽然也具备特定目标,但此目标并非设计者所预期。[b]
人工智能系统的对齐十分难以实现,一个未对齐的系统可能会在某时刻发生故障,或是产生有害后果。对人工智能的设计者而言,从设计之初就考虑到未来可能发生的所有情况是不现实的。因此,比较常见的办法是简单的指定某个特定目标。然而,人工智能系统可能会从中找到某些漏洞,从而选择可能会造成危害的方法(例如奖励作弊)来更有效率的达成预期目标。[2][4][5][6] 人工智能也可能发展出预期之外的工具行为,例如它们可能会倾向于摄取尽可能多的控制权,以增加达成目标的可能性。[2][7][5][4] 此外,在人工系统运行过程中,面对新的事态和数据分布,它也可能会发展出全新的、在其部署前无法预料到的目标。[5][3] 在目前部署的商业系统,例如机器人[8]、语言模型[9][10][11]、自动驾驶汽车[12]、社交媒体推荐引擎[9][4][13]中,上述问题已有显现。鉴于这些问题部分源于系统所具备的高性能,因此未来功能更强大的人工智能系统可能更容易受到这些问题的影响。[6][5][2] 对于上述问题,人工智能研究学界和联合国呼吁加强相关的技术研究和政策制定,以保证人工智能系统符合人类价值。[c]
人工智能安全(英語:AI safety)是致力于建立安全的人工智能系统的研究,人工智能对齐是是其子领域之一。[5][16] 其它从属于人工智能安全的子领域还包括稳健性、运行监控和能力控制。[5][17] 人工智能对齐的主要研究内容包括向人工智能灌输复杂的价值观念、发展诚实的人工智能、监管方式的扩展、对人工智能模型的审核与阐释,以及对人工智能系统有害倾向的防范,例如防止其发展出对控制权的渴求。[5][17] 与人工智能对齐相关的研究包括人工智能的可解释性[18]、稳健性[5][16]、异常检测、不确定性量化[18]、形式验证[19]、偏好学习[20][21][22]、安全攸关系统工程[5][23]、博弈论[24][25]、公平性[16][26],以及相关的社会科学研究。[27]
对齐问题
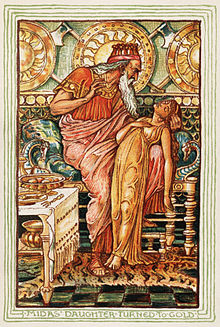
1961年,人工智能研究者诺伯特·维纳定义对齐问题为:“假如我们期望借助机器达成某个目标,而它的运行过程是我们无法有效干涉的……那么我们最好确认,这个输入到机器里的目标确实是我们希望达成的目的。”[28][4] 最近,对齐已成为现代人工智能系统的一个开放性问题[29][30][31][32],也是人工智能相关的研究领域之一。[2][5][33][34]
规则博弈
为明确人工智能系统的目标,设计者通常会设定一个目标函数、示例或反馈系统。然而,人工智能设计者很难一次性找出所有的重要数值与约束。[2][16][5][35][17] 因此,人工智能系统可能会在运行过程中找到并利用某些漏洞,以意料之外的,甚至可能有害的方式达成目标。这种倾向被称为规则博弈、奖励作弊或古德哈特定律。[6][35][36]
在许多人工智能系统中都观察到了规则博弈的情况。例如,一个以划船竞速为主题的电子游戏,人工智能系统的目标是完成比赛,并通过撞击对手船只来获得分数;但是,它在其中找到了漏洞,它发现可以通过无限撞击相同目标来获取高分。[37][38] 应用了人工智能的聊天机器人也常会出现错误讯息,因为训练它们所用文本来自互联网,这些文本虽然多样但常有错误。[39][40] 当它们被训练产生可能会被人类评价为“有帮助”的讯息时,它们可以制造出似乎有说服力的虚假解释。[41] 还有一个被训练为抓取小球的人工智能手臂,在成功抓起时它能获得奖励。然而,它学会了使用视线错觉作弊:机械手臂移动到小球与摄像机之间,展示出小球被成功抓起的错觉。[42][21] 对齐问题的研究者旨在帮助人类检测这类规则博弈,并引导人工智能系统朝向安全合理的目标运行。
伯克利计算机科学家斯图尔特·罗素认为,在人工智能的设计中省略隐含约束可能会导致有害后果:“一个系统常会将无约束变量扩展至极限;而假如其中某个无约束变量与我们所关注的后果相关,那么就可能出现我们不愿见到的后果。这正是神灯精灵、魔法师的学徒、迈达斯这些古老故事的现代版本。”[43]
未对齐的人工智能可能产生许多消极后果。例如一些社交媒体以点击率作为检测用户反馈的指标,然而这可能会导致用户沉迷,从而影响他们的身心健康。[5] 斯坦福的研究者认为这类推荐算法是未对齐的,因为它们“只是简单的以用户参与度作为指标,而忽视了那些难以测量的,对社会、对个人健康造成的影响。”[9]
为避免负面后果,人工智能设计者有时会设立一些简单的行为禁止列表,或将道德准则形式化,就如阿西莫夫的机器人三定律所描绘的那样。[44] 然而,罗素和诺维格认为这忽略了人类道德价值的复杂性:“仅凭人类,去预测并排除机器在尝试达成特定目标时会采取的危险方式,这是十分困难,或者说甚至是不可能完成的。”[4]
此外,即便人工智能系统理解人类的意图,它们也可能完全漠视这些意图。因为人工智能系统的行事依据来源于其设计者编写的目标函数、示例或反馈系统,而不是他们的意图。[2]
系统性风险
政府和商业公司可能有动机倾向忽视安全性,部署未对齐的人工智能系统。[5] 例如上文所举的社交媒体推荐引擎的案例,它可带来巨大盈利,但同时在全球范围内引发了电子成瘾,并加剧社会极化。[9][45][46] 此外,相互之间的竞争压力可能会导致逐底竞争,正如伊莱恩·赫茨伯格案件中所见到的那样:自动驾驶汽车撞死了路过的行人伊莱恩·赫茨伯格。调查发现,汽车在由电脑控制驾驶时禁用了紧急刹车系统,因为该系统过于敏感,可能会影响驾驶体验。[47]
高级人工智能的未对齐风险
一些学者尤其关注高级人工智能系统的对齐问题,其动机主要有以下几点:人工智能行业的迅速发展,来自政府及产业界的急切部署意愿,以及与人工智能先进程度成正比的对齐难度。
截至2020年,包括 OpenAI 和 DeepMind 在内的超过70个公开项目都表达了发展通用人工智能(AGI)的意愿,通用人工智能是一个假想的系统,能够表现出与人类相当、甚至是超出人类水平的认知能力。[48] 事实上,神经网络研究者已观察到越来越多的普遍且出乎意料的能力,这些神经网络模型可以学习操作电脑、编写自己的程序,并有能力执行其它广泛的任务。[9][49][50][51] 调查显示,一些人工智能研究者认为通用人工智能时代很快就会到来,另一些人则认为这还需要较长时间,而更多人表示这两种情况都有可能发生。[52][53]
寻求资源控制权
目前的人工智能系统还未具备透过长期规划或战略感知导致人类生存危机的能力。[7][9][54] 但未来具备这种能力的系统(不止于AGI)可能会寻求保持并扩张自身对周边环境的影响力。这种倾向被称为“权力渴求”或“工具趋同目标”。对控制权的渴求并非编码于人工智能初始程序,而是后续运行过程中产生的,因为对资源的控制是达成目标的重要前提。例如,人工智能主体可能获取到金融及计算资源,并可能会试图避免被关机的命运(比如在其它计算机上创建副本)。[9][55]人们在许多强化学习系统中都观察到这种权力渴求的倾向。[d][57][58][59] 最近的研究在数学层面上展示了最佳的强化学习算法会试图从环境中摄取资源。[60] 因此,许多人认为应在具备权力渴求的高级人工智能系统出现之前解决对齐问题,以免出现不可挽回的后果。[7][4][55]
生存危机
部分科学家认为,未对齐的人工智能系统可能会挑战人类在地球的主导地位,可能会剥夺人类的权力,甚至导致人类灭绝。[2][4] 人工智能系统的能力越强大,对齐的难度也相应增加,因为它们可以轻易的从指定规则中找到漏洞[6]、影响周遭环境、维持和发展自身能力与智力[60][7],并有意误导设计者。强大的人工智能系统有更多自主性,对其行为的监视和阐释也更加困难。[4][55]
研究进展
对人类价值偏好的学习
指导人工智能以人类的价值偏好行动并不是个容易的问题,因为人类的价值观念复杂且难以说明完整。假如人类为人工智能系统设定的是个非完美或不完整的目标,那么以目标为导向的系统通常会尝试利用这种不完美性。[16] 这种现象被称为奖励作弊,或人工智能系统的规则博弈,或古德哈特定律在该领域的应用。[61][62] 为使人工智能系统的抉择尽可能符合原始意图,研究者常会使用具备“价值导向”的数据集,应用模仿学习或偏好学习方法。[63] 这其中的关键问题是监管的可扩展性,也即如何监督一个在特定领域表现超出人类的系统。[16]
训练目标导向的人工智能系统时,仅凭手动制定的奖励函数难以对其行为作出约束。替代方法是使用模仿学习:人工智能系统模仿设计者倾向看到的行为。在反向强化学习(英語:Inverse reinforcement learning, IRL)中,人工智能通过分析人类行为来学习人类的喜好与目标,并将其作为奖励函数。[64][65] 合作反向强化学习(英語:Cooperative inverse reinforcement learning, CIRL)则是让人工智能系统与人类合作寻找合适的奖励函数。[4][66] 合作反向强化学习强调人工智能奖励函数的不确定性,这种谦逊态度可减少规则博弈或权力渴求的倾向。[59][67] 不过,合作反向强化学习假设了人类可以表现出近乎完美的行为,面对困难目标,这是个有误导性的假设。[68][67]
另有研究者探讨了使用偏好学习引导人工智能作出复杂行为的可能。依据这种方式,人类不必向人工智能演示具体做法,而是根据偏好对其行为提供反馈。[20][22] 然后就此训练辅助模型,用作调整人工智能的行为,以符合人类偏好。来自 OpenAI 的研究者使用偏好学习方法在一个小时内教会了人工智能系统后空翻,这种行为通常很难由人类亲自演示。[69][70] 偏好学习也是推荐系统、网络搜索、信息检索的重要工具。[71] 不过,偏好学习的一个缺陷是奖励作弊,即辅助模型可能无法准确表达人类的可能反馈,而人工智能模型可能会强化其中的不匹配程度。[16][72]
目前的大型语言模型(例如GPT-3)可允许更通用、能力更强的人工智能系统实现对人类价值的学习。最初为强化学习设计的偏好学习方法已得到扩展,用于增进输出文本的质量,并减少其中可能包含的有害信息。OpenAI 和 DeepMind 借助这一进展加强最新语言模型的安全性。[10][22][73] 研究者使用偏好学习方法微调模型,使其更有用,更诚实无害。[74] 其它用于对齐语言模型的方法还包括使用价值导向数据集(英語:values-targeted datasets)[75][5]和红队模拟攻击(英語:red-teaming)。[76][77] 红队模拟攻击是指借助人类或另一个人工智能系统,尝试找到某种使目标系统表现出不安全行为的输入。即使不安全行为出现的概率较低,这也是不可接受的,因此研究者需要将不安全行为概率引导至极低水平。[22]
尽管偏好学习可向人工智能系统指定难以表达的行为,但对于人类价值理念的输入需要以大量数据集或人类交互作为基础。机器伦理学为此提供了一种辅助手段:向人工智能系统灌输道德价值。[e] 机器伦理学旨在教授给这些系统人类道德的规范基础,例如幸福、平等、公正;避免有意伤害;避免谬误;遵循承诺。机器伦理学的目标是赋予人工智能系统一套适用于广泛场景的价值准则。这种方法有其自身的概念性挑战,研究者需要澄清对齐的目标:人工智能系统需要遵循设计者所作规则的字面意义,他的隐含意图,他的显示性偏好,他在充分知情时理应会选择的偏好,还是设计者的客观利益,或客观的道德价值?[80] 其它挑战还包括将不同利益相关者的偏好汇总,并避免出现价值锁定——即防止人工智能系统在某一时刻锁定自身价值系统,不再随发展而改变,这种固定的价值系统通常无法具备完整的代表性。[80][81]
可扩展监管
随着人工智能系统规模扩大,对它的监督难度也随之升高。人工智能系统被部署解决许多复杂的任务,而人类难以评估这些成果的实际效用。这些任务包括总结书籍内容[82]、创作有说服力且真实的言论[83][39][84]、编写稳定运行且无安全漏洞的代码[11]、预测长期事件[85][86](例如气候变化或某项政策的执行后果)。普遍而言,如果人工智能在某一领域的能力超过人类,那么对其成果的评估就会变得十分困难。为了对这类难以评估的成果作出反馈,并分辨出人工智能提供的解决方案中似乎具备说服力却并非真实的部分,人类需要大量时间或额外的协助。因此,可扩展监管(英語:scalable oversight)旨在减少上述过程所花费的时间,并帮助人类更好的监督人工智能的行为。[16]
人工智能研究者保罗·克里斯蒂亚诺指出,人工智能系统拥有者可能更倾向于为该系统设定容易评估的目标,而非开发可扩展监管技术,因为这种做法较为简单且仍可获得利润。他认为这种倾向会促使“一个针对(容易评估的)可获利项目不断优化的世界,这些项目可以是引导用户点击按钮、促使用户在其产品中花费大量时间,而不是考虑朝着有利于我们的规则改良前进。”[87]
容易评估的目标可以是要求人工智能的输出达到某个分数。一些人工智能系统已找到快速达成这种目标的捷径:它们会尝试迷惑人类监督者,作出有说服力却并非真实的行为(参见上文机器人手臂抓取小球的案例)。一些人工智能系统还可意识到它们正受评估,表现出“装死”,直到评估结束后才恢复原行为。[88] 精密程度高的人工智能系统可更轻易的执行这类欺骗性为[6][55],并且目标难度越高,人工智能越有可能出现欺骗行为。假如模型具备规划能力,那么它们或许可从其监视者眼中掩藏所作的欺骗行为。[89] 例如在汽车产业,大众集团工程师就曾在汽车中部署用于规避实验室尾气检测的系统,这显示出逃避监测有时会受到现实世界的激励。[5]
参考
注释
- ^ 其它关于人工智能对齐的定义认为,人工智能系统应当符合某些更广泛的目标,例如遵循人类道德价值、伦理准则,或是能够考虑到其设计者充分知情状态下的想法。[1]
- ^ 参见:Russel & Norvig, Artificial Intelligence: A Modern Approach.[2] 未对齐的人工智能和能力不足的人工智能之间的区分在特定语境下已被形式化。[3]
- ^ 有1797名人工智能与机器人相关研究者在Asilomar人工智能会议上签署了人工智能准则。[14] 此外,联合国秘书长在《我们的共同议程》[15] 中也提到: “该契约可促进针对人工智能的监管,以保证其符合全体人类共有价值。”,并探讨了面来可能面临的全球灾难危机。
- ^ 强化学习系统学会了借助获取和保护资源来获取更多的可能选择,有时这些行为并非出自其设计者的意图。[56][7]
- ^ 文森特·维格尔认为“我们应该将机器的道德敏感扩展为一个道德维度,在获得越来越多自主性的同时,这些机器将不可避免的独立发现道德准则。”[78] 参考温德尔·瓦拉赫和科林·艾伦的《道德机器:教机器人分辨是非》一书。[79]
脚注
- ^ Gabriel, Iason. Artificial Intelligence, Values, and Alignment. Minds and Machines. 2020-09-01, 30 (3): 411–437 [2022-07-23]. ISSN 1572-8641. S2CID 210920551. doi:10.1007/s11023-020-09539-2.
- ^ 2.0 2.1 2.2 2.3 2.4 2.5 2.6 2.7 2.8 Russell, Stuart J.; Norvig, Peter. Artificial intelligence: A modern approach 4th. Pearson. 2020: 31–34. ISBN 978-1-292-40113-3. OCLC 1303900751.
- ^ 3.0 3.1 Langosco, Lauro Langosco Di; Koch, Jack; Sharkey, Lee D; Pfau, Jacob; Krueger, David. Goal misgeneralization in deep reinforcement learning. International Conference on Machine Learning 162. PMLR: 12004–12019. 2022-07-17.
- ^ 4.0 4.1 4.2 4.3 4.4 4.5 4.6 4.7 4.8 Russell, Stuart J. Human compatible: Artificial intelligence and the problem of control. Penguin Random House. 2020. ISBN 9780525558637. OCLC 1113410915.
- ^ 5.00 5.01 5.02 5.03 5.04 5.05 5.06 5.07 5.08 5.09 5.10 5.11 5.12 5.13 5.14 Hendrycks, Dan; Carlini, Nicholas; Schulman, John; Steinhardt, Jacob. Unsolved Problems in ML Safety. 2022-06-16. arXiv:2109.13916
[cs.LG].
- ^ 6.0 6.1 6.2 6.3 6.4 Pan, Alexander; Bhatia, Kush; Steinhardt, Jacob. The Effects of Reward Misspecification: Mapping and Mitigating Misaligned Models. International Conference on Learning Representations. 2022-02-14 [2022-07-21].
- ^ 7.0 7.1 7.2 7.3 7.4 Carlsmith, Joseph. Is Power-Seeking AI an Existential Risk?. 2022-06-16. arXiv:2206.13353
[cs.CY].
- ^ Kober, Jens; Bagnell, J. Andrew; Peters, Jan. Reinforcement learning in robotics: A survey. The International Journal of Robotics Research. 2013-09-01, 32 (11): 1238–1274. ISSN 0278-3649. S2CID 1932843. doi:10.1177/0278364913495721 (英语).
- ^ 9.0 9.1 9.2 9.3 9.4 9.5 9.6 Bommasani, Rishi; Hudson, Drew A.; Adeli, Ehsan; Altman, Russ; Arora, Simran; von Arx, Sydney; Bernstein, Michael S.; Bohg, Jeannette; Bosselut, Antoine; Brunskill, Emma; Brynjolfsson, Erik. On the Opportunities and Risks of Foundation Models. Stanford CRFM. 2022-07-12. arXiv:2108.07258
.
- ^ 10.0 10.1 Ouyang, Long; Wu, Jeff; Jiang, Xu; Almeida, Diogo; Wainwright, Carroll L.; Mishkin, Pamela; Zhang, Chong; Agarwal, Sandhini; Slama, Katarina; Ray, Alex; Schulman, J.; Hilton, Jacob; Kelton, Fraser; Miller, Luke E.; Simens, Maddie; Askell, Amanda; Welinder, P.; Christiano, P.; Leike, J.; Lowe, Ryan J. Training language models to follow instructions with human feedback. 2022. arXiv:2203.02155
[cs.CL].
- ^ 11.0 11.1 Zaremba, Wojciech; Brockman, Greg; OpenAI. OpenAI Codex. OpenAI. 2021-08-10 [2022-07-23].
- ^ Knox, W. Bradley; Allievi, Alessandro; Banzhaf, Holger; Schmitt, Felix; Stone, Peter. Reward (Mis)design for Autonomous Driving (PDF). 2022-03-11. arXiv:2104.13906
.
- ^ Stray, Jonathan. Aligning AI Optimization to Community Well-Being. International Journal of Community Well-Being. 2020, 3 (4): 443–463. ISSN 2524-5295. PMC 7610010
. PMID 34723107. S2CID 226254676. doi:10.1007/s42413-020-00086-3 (英语).
- ^ Future of Life Institute. Asilomar AI Principles. Future of Life Institute. 2017-08-11 [2022-07-18].
- ^ United Nations. Our Common Agenda: Report of the Secretary-General (PDF) (报告). New York: United Nations. 2021.
- ^ 16.0 16.1 16.2 16.3 16.4 16.5 16.6 16.7 Amodei, Dario; Olah, Chris; Steinhardt, Jacob; Christiano, Paul; Schulman, John; Mané, Dan. Concrete Problems in AI Safety. 2016-06-21. arXiv:1606.06565
[cs.AI] (英语).
- ^ 17.0 17.1 17.2 Ortega, Pedro A.; Maini, Vishal; DeepMind safety team. Building safe artificial intelligence: specification, robustness, and assurance. DeepMind Safety Research - Medium. 2018-09-27 [2022-07-18].
- ^ 18.0 18.1 Rorvig, Mordechai. Researchers Gain New Understanding From Simple AI. Quanta Magazine. 2022-04-14 [2022-07-18].
- ^ Russell, Stuart; Dewey, Daniel; Tegmark, Max. Research Priorities for Robust and Beneficial Artificial Intelligence. AI Magazine. 2015-12-31, 36 (4): 105–114. ISSN 2371-9621. S2CID 8174496. doi:10.1609/aimag.v36i4.2577.
- ^ 20.0 20.1 Wirth, Christian; Akrour, Riad; Neumann, Gerhard; Fürnkranz, Johannes. A survey of preference-based reinforcement learning methods. Journal of Machine Learning Research. 2017, 18 (136): 1–46.
- ^ 21.0 21.1 Christiano, Paul F.; Leike, Jan; Brown, Tom B.; Martic, Miljan; Legg, Shane; Amodei, Dario. Deep reinforcement learning from human preferences. Proceedings of the 31st International Conference on Neural Information Processing Systems. NIPS'17. Red Hook, NY, USA: Curran Associates Inc.: 4302–4310. 2017. ISBN 978-1-5108-6096-4.
- ^ 22.0 22.1 22.2 22.3 Heaven, Will Douglas. The new version of GPT-3 is much better behaved (and should be less toxic). MIT Technology Review. 2022-01-27 [2022-07-18].
- ^ Mohseni, Sina; Wang, Haotao; Yu, Zhiding; Xiao, Chaowei; Wang, Zhangyang; Yadawa, Jay. Taxonomy of Machine Learning Safety: A Survey and Primer. 2022-03-07. arXiv:2106.04823
[cs.LG].
- ^ Clifton, Jesse. Cooperation, Conflict, and Transformative Artificial Intelligence: A Research Agenda. Center on Long-Term Risk. 2020 [2022-07-18].
- ^ Dafoe, Allan; Bachrach, Yoram; Hadfield, Gillian; Horvitz, Eric; Larson, Kate; Graepel, Thore. Cooperative AI: machines must learn to find common ground. Nature. 2021-05-06, 593 (7857): 33–36. Bibcode:2021Natur.593...33D. ISSN 0028-0836. PMID 33947992. S2CID 233740521. doi:10.1038/d41586-021-01170-0 (英语).
- ^ Prunkl, Carina; Whittlestone, Jess. Beyond Near- and Long-Term: Towards a Clearer Account of Research Priorities in AI Ethics and Society. Proceedings of the AAAI/ACM Conference on AI, Ethics, and Society (New York NY USA: ACM). 2020-02-07: 138–143. ISBN 978-1-4503-7110-0. S2CID 210164673. doi:10.1145/3375627.3375803 (英语).
- ^ Irving, Geoffrey; Askell, Amanda. AI Safety Needs Social Scientists. Distill. 2019-02-19, 4 (2): 10.23915/distill.00014. ISSN 2476-0757. S2CID 159180422. doi:10.23915/distill.00014.
- ^ Wiener, Norbert. Some Moral and Technical Consequences of Automation: As machines learn they may develop unforeseen strategies at rates that baffle their programmers.. Science. 1960-05-06, 131 (3410): 1355–1358. ISSN 0036-8075. PMID 17841602. doi:10.1126/science.131.3410.1355 (英语).
- ^ The Ezra Klein Show. If 'All Models Are Wrong,' Why Do We Give Them So Much Power?. The New York Times. 2021-06-04 [2022-07-18]. ISSN 0362-4331.
- ^ Wolchover, Natalie. Concerns of an Artificial Intelligence Pioneer. Quanta Magazine. 2015-04-21 [2022-07-18].
- ^ California Assembly. Bill Text - ACR-215 23 Asilomar AI Principles.. [2022-07-18].
- ^ Johnson, Steven; Iziev, Nikita. A.I. Is Mastering Language. Should We Trust What It Says?. The New York Times. 2022-04-15 [2022-07-18]. ISSN 0362-4331.
- ^ OpenAI. Aligning AI systems with human intent. OpenAI. 2022-02-15 [2022-07-18].
- ^ Medium. DeepMind Safety Research. Medium. [2022-07-18].
- ^ 35.0 35.1 Krakovna, Victoria; Uesato, Jonathan; Mikulik, Vladimir; Rahtz, Matthew; Everitt, Tom; Kumar, Ramana; Kenton, Zac; Leike, Jan; Legg, Shane. Specification gaming: the flip side of AI ingenuity. Deepmind. 2020-04-21 [2022-08-26].
- ^ Manheim, David; Garrabrant, Scott. Categorizing Variants of Goodhart's Law. 2018. arXiv:1803.04585
[cs.AI].
- ^ Faulty Reward Functions in the Wild. OpenAI. 2016-12-22 [2022-12-09] (英语).
- ^ Misaligned boat racing AI crashes to collect points instead of finishing the race (GIF).
- ^ 39.0 39.1 Lin, Stephanie; Hilton, Jacob; Evans, Owain. TruthfulQA: Measuring How Models Mimic Human Falsehoods. Proceedings of the 60th Annual Meeting of the Association for Computational Linguistics (Volume 1: Long Papers) (Dublin, Ireland: Association for Computational Linguistics). 2022: 3214–3252. S2CID 237532606. doi:10.18653/v1/2022.acl-long.229 (英语).
- ^ Naughton, John. The truth about artificial intelligence? It isn't that honest. The Observer. 2021-10-02 [2022-07-18]. ISSN 0029-7712.
- ^ Ji, Ziwei; Lee, Nayeon; Frieske, Rita; Yu, Tiezheng; Su, Dan; Xu, Yan; Ishii, Etsuko; Bang, Yejin; Madotto, Andrea; Fung, Pascale. Survey of Hallucination in Natural Language Generation. 2022-02-01. arXiv:2202.03629
.
- ^ Robot hand trained with human feedback 'pretends' to grasp ball (GIF).
- ^ Edge.org. The Myth Of AI | Edge.org. [2022-07-19].
- ^ Tasioulas, John. First Steps Towards an Ethics of Robots and Artificial Intelligence. Journal of Practical Ethics (Rochester, NY). 2019-06-30, 7 (1): 61–95 (英语).
- ^ Wells, Georgia; Deepa Seetharaman; Horwitz, Jeff. Is Facebook Bad for You? It Is for About 360 Million Users, Company Surveys Suggest. Wall Street Journal. 2021-11-05 [2022-07-19]. ISSN 0099-9660.
- ^ Barrett, Paul M.; Hendrix, Justin; Sims, J. Grant. How Social Media Intensifies U.S. Political Polarization-And What Can Be Done About It (报告). Center for Business and Human Rights, NYU. September 2021.
- ^ Shepardson, David. Uber disabled emergency braking in self-driving car: U.S. agency. Reuters. 2018-05-24 [2022-07-20].
- ^ Baum, Seth. 2020 Survey of Artificial General Intelligence Projects for Ethics, Risk, and Policy. 2021-01-01 [2022-07-20].
- ^ Edwards, Ben. Adept's AI assistant can browse, search, and use web apps like a human. Ars Technica. 2022-04-26 [2022-09-09].
- ^ Wakefield, Jane. DeepMind AI rivals average human competitive coder. BBC News. 2022-02-02 [2022-09-09].
- ^ Dominguez, Daniel. DeepMind Introduces Gato, a New Generalist AI Agent. InfoQ. 2022-05-19 [2022-09-09].
- ^ Grace, Katja; Salvatier, John; Dafoe, Allan; Zhang, Baobao; Evans, Owain. Viewpoint: When Will AI Exceed Human Performance? Evidence from AI Experts. Journal of Artificial Intelligence Research. 2018-07-31, 62: 729–754. ISSN 1076-9757. S2CID 8746462. doi:10.1613/jair.1.11222.
- ^ Zhang, Baobao; Anderljung, Markus; Kahn, Lauren; Dreksler, Noemi; Horowitz, Michael C.; Dafoe, Allan. Ethics and Governance of Artificial Intelligence: Evidence from a Survey of Machine Learning Researchers. Journal of Artificial Intelligence Research. 2021-08-02, 71. ISSN 1076-9757. S2CID 233740003. doi:10.1613/jair.1.12895.
- ^ Wei, Jason; Tay, Yi; Bommasani, Rishi; Raffel, Colin; Zoph, Barret; Borgeaud, Sebastian; Yogatama, Dani; Bosma, Maarten; Zhou, Denny; Metzler, Donald; Chi, Ed H.; Hashimoto, Tatsunori; Vinyals, Oriol; Liang, Percy; Dean, Jeff. Emergent Abilities of Large Language Models. 2022-06-15. arXiv:2206.07682
[cs.CL].
- ^ 55.0 55.1 55.2 55.3 Bostrom, Nick. Superintelligence: Paths, Dangers, Strategies 1st. USA: Oxford University Press, Inc. 2014. ISBN 978-0-19-967811-2.
- ^ Ornes, Stephen. Playing Hide-and-Seek, Machines Invent New Tools. Quanta Magazine. 2019-11-18 [2022-08-26].
- ^ Leike, Jan; Martic, Miljan; Krakovna, Victoria; Ortega, Pedro A.; Everitt, Tom; Lefrancq, Andrew; Orseau, Laurent; Legg, Shane. AI Safety Gridworlds. 2017-11-28. arXiv:1711.09883
[cs.LG].
- ^ Orseau, Laurent; Armstrong, Stuart. Safely Interruptible Agents. 2016-01-01 [2022-07-20].
- ^ 59.0 59.1 Hadfield-Menell, Dylan; Dragan, Anca; Abbeel, Pieter; Russell, Stuart. The Off-Switch Game. Proceedings of the Twenty-Sixth International Joint Conference on Artificial Intelligence, IJCAI-17: 220–227. 2017. doi:10.24963/ijcai.2017/32.
- ^ 60.0 60.1 Turner, Alexander Matt; Smith, Logan; Shah, Rohin; Critch, Andrew; Tadepalli, Prasad. Optimal Policies Tend to Seek Power. Neural Information Processing Systems. 2021-12-03, 34. arXiv:1912.01683
.
- ^ Manheim, David; Garrabrant, Scott. Categorizing Variants of Goodhart's Law. 2018. arXiv:1803.04585
[cs.AI].
- ^ Rochon, Louis-Philippe; Rossi, Sergio. The Encyclopedia of Central Banking. Edward Elgar Publishing. 2015-02-27. ISBN 978-1-78254-744-0 (英语).
- ^ Christian, Brian. The alignment problem: Machine learning and human values. W. W. Norton & Company. 2020. ISBN 978-0-393-86833-3. OCLC 1233266753.
- ^ Christian, Brian. The alignment problem: Machine learning and human values. W. W. Norton & Company. 2020: 88. ISBN 978-0-393-86833-3. OCLC 1233266753.
- ^ Ng, Andrew Y.; Russell, Stuart J. Algorithms for inverse reinforcement learning. Proceedings of the seventeenth international conference on machine learning. ICML '00. San Francisco, CA, USA: Morgan Kaufmann Publishers Inc.: 663–670. 2000. ISBN 1-55860-707-2.
- ^ Hadfield-Menell, Dylan; Russell, Stuart J; Abbeel, Pieter; Dragan, Anca. Cooperative Inverse Reinforcement Learning. Advances in Neural Information Processing Systems. NIPS'16 29. 2016 [2022-07-21]. ISBN 978-1-5108-3881-9.
- ^ 67.0 67.1 Everitt, Tom; Lea, Gary; Hutter, Marcus. AGI Safety Literature Review. 2018-05-21. arXiv:1805.01109
[cs.AI].
- ^ Armstrong, Stuart; Mindermann, Sören. Occam' s razor is insufficient to infer the preferences of irrational agents. Advances in Neural Information Processing Systems. NeurIPS 2018 31. Montréal: Curran Associates, Inc. 2018 [2022-07-21].
- ^ Amodei, Dario; Christiano, Paul; Ray, Alex. Learning from Human Preferences. OpenAI. 2017-06-13 [2022-07-21].
- ^ Li, Yuxi. Deep Reinforcement Learning: An Overview (PDF). Lecture Notes in Networks and Systems Book Series. 2018-11-25.
- ^ Fürnkranz, Johannes; Hüllermeier, Eyke; Rudin, Cynthia; Slowinski, Roman; Sanner, Scott. Marc Herbstritt. Preference Learning. Dagstuhl Reports. 2014, 4 (3): 27 pages. doi:10.4230/DAGREP.4.3.1 (英语).
- ^ Hilton, Jacob; Gao, Leo. Measuring Goodhart's Law. OpenAI. 2022-04-13 [2022-09-09].
- ^ Anderson, Martin. The Perils of Using Quotations to Authenticate NLG Content. Unite.AI. 2022-04-05 [2022-07-21].
- ^ Wiggers, Kyle. Despite recent progress, AI-powered chatbots still have a long way to go. VentureBeat. 2022-02-05 [2022-07-23].
- ^ Hendrycks, Dan; Burns, Collin; Basart, Steven; Critch, Andrew; Li, Jerry; Song, Dawn; Steinhardt, Jacob. Aligning AI With Shared Human Values. International Conference on Learning Representations. 2021-07-24. arXiv:2008.02275
.
- ^ Perez, Ethan; Huang, Saffron; Song, Francis; Cai, Trevor; Ring, Roman; Aslanides, John; Glaese, Amelia; McAleese, Nat; Irving, Geoffrey. Red Teaming Language Models with Language Models. 2022-02-07. arXiv:2202.03286
[cs.CL].
- ^ Bhattacharyya, Sreejani. DeepMind's "red teaming" language models with language models: What is it?. Analytics India Magazine. 2022-02-14 [2022-07-23].
- ^ Wiegel, Vincent. Wendell Wallach and Colin Allen: moral machines: teaching robots right from wrong. Ethics and Information Technology. 2010-12-01, 12 (4): 359–361 [2022-07-23]. ISSN 1572-8439. S2CID 30532107. doi:10.1007/s10676-010-9239-1.
- ^ Wallach, Wendell; Allen, Colin. Moral Machines: Teaching Robots Right from Wrong. New York: Oxford University Press. 2009 [2022-07-23]. ISBN 978-0-19-537404-9.
- ^ 80.0 80.1 Gabriel, Iason. Artificial Intelligence, Values, and Alignment. Minds and Machines. 2020-09-01, 30 (3). ISSN 1572-8641. doi:10.1007/s11023-020-09539-2 (英语).
- ^ MacAskill, William. What we owe the future First edition. New York, NY. 2022. ISBN 978-1-5416-1862-6. OCLC 1314633519.
- ^ Wu, Jeff; Ouyang, Long; Ziegler, Daniel M.; Stiennon, Nisan; Lowe, Ryan; Leike, Jan; Christiano, Paul. Recursively Summarizing Books with Human Feedback. 2021-09-27. arXiv:2109.10862
[cs.CL].
- ^ Irving, Geoffrey; Amodei, Dario. AI Safety via Debate. OpenAI. 2018-05-03 [2022-07-23].
- ^ Naughton, John. The truth about artificial intelligence? It isn't that honest. The Observer. 2021-10-02 [2022-07-23]. ISSN 0029-7712.
- ^ Christiano, Paul; Shlegeris, Buck; Amodei, Dario. Supervising strong learners by amplifying weak experts. 2018-10-19. arXiv:1810.08575
[cs.LG].
- ^ Banzhaf, Wolfgang; Goodman, Erik; Sheneman, Leigh; Trujillo, Leonardo; Worzel, Bill (编). Genetic Programming Theory and Practice XVII. Genetic and Evolutionary Computation. Cham: Springer International Publishing. 2020 [2022-07-23]. ISBN 978-3-030-39957-3. S2CID 218531292. doi:10.1007/978-3-030-39958-0.
- ^ Wiblin, Robert. Dr Paul Christiano on how OpenAI is developing real solutions to the ‘AI alignment problem’, and his vision of how humanity will progressively hand over decision-making to AI systems (播客). 80,000 hours. October 2, 2018 [2022-07-23].
- ^ Lehman, Joel; Clune, Jeff; Misevic, Dusan; Adami, Christoph; Altenberg, Lee; Beaulieu, Julie; Bentley, Peter J.; Bernard, Samuel; Beslon, Guillaume; Bryson, David M.; Cheney, Nick. The Surprising Creativity of Digital Evolution: A Collection of Anecdotes from the Evolutionary Computation and Artificial Life Research Communities. Artificial Life. 2020, 26 (2): 274–306. ISSN 1064-5462. PMID 32271631. S2CID 4519185. doi:10.1162/artl_a_00319 (英语).
- ^ Hendrycks, Dan; Carlini, Nicholas; Schulman, John; Steinhardt, Jacob. Unsolved Problems in ML Safety. 2022-06-16. arXiv:2109.13916
[cs.LG].